The Kadanoff’s block-spin method gives a nice and simple physical picture for renormalization group (RG) transformations. Combined with Migdal’s bond-moving trick, it serves as a first approximation to estimate phase diagram and critical exponents. However, it is difficult to improve the estimations systematically.
Modern tensor-network-based methods open up new possibilities for RG transformations. They generalize the conventional real-space RG transformations and are more versatile and systematically improvable. They are most convenient for calculations of free energy.
In this paper [1], we fully exploit the RG interpretation of these tensor-network-based methods and show a way to carry out the textbook RG prescription in tensor-network language: identify a fixed point, linearize the RG equation around this fixed point, and diagonalize this linearized RG equation to obtain scaling dimensions, to which the critical exponents are related. The proposed method works equally well compared with the current bread-and-butter tensor method for extracting scaling dimensions in the context of the 2D classical Ising model. The advantage is its potential applications to 3D systems, where the bread-and-butter method is inapplicable.
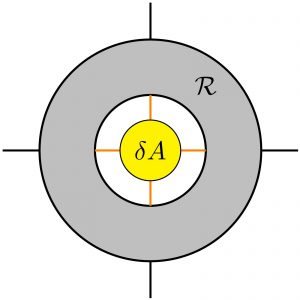
References
- X. Lyu, R.Q.G. Xu, and N. Kawashima, Phys. Rev. Research 3, 023048 (2021)