We proposed [1] a new method for constructing a generative model based on a tensor network (TN) and demonstrated its effectiveness. In most cases, generative models are based on neural networks, and optimization of the network structure has not been fully investigated. We considered a Born machine that uses the correspondence between wave functions expressed as TNs and probability distributions represented as their amplitude. Based on this correspondence, we proposed a generative model construction method (adaptive tensor tree, ATT) that optimizes the network structure, and demonstrated its effectiveness. The structure optimization was performed to minimize the mutual information carried by the tree branches. In other words, if the mutual information of each branch decreases when the surrounding branches are rearranged, the rearrangement is performed. As an example, when a generative model was constructed using ATT from data on stock price rise and fall patterns, it was observed that the correlation between stocks was naturally reflected in the network structure as learning progressed. The figure shows a tree structure generated by a Born machine based on an adaptive tensor tree (ATT) learning about 10 years of rise and fall patterns of the S&P 500. Each dot represents a company, and the color changes depending on the industry category (called “sector”). Although sector information was not provided as learning information for ATT, it can be seen that it has “discovered” that there is a close relationship between the sector and the branch structure. Because ATT can build a generative model for any sample, it is expected to be useful for elucidating various correlation structures that were previously difficult to capture, and may provide a new framework for building AI.
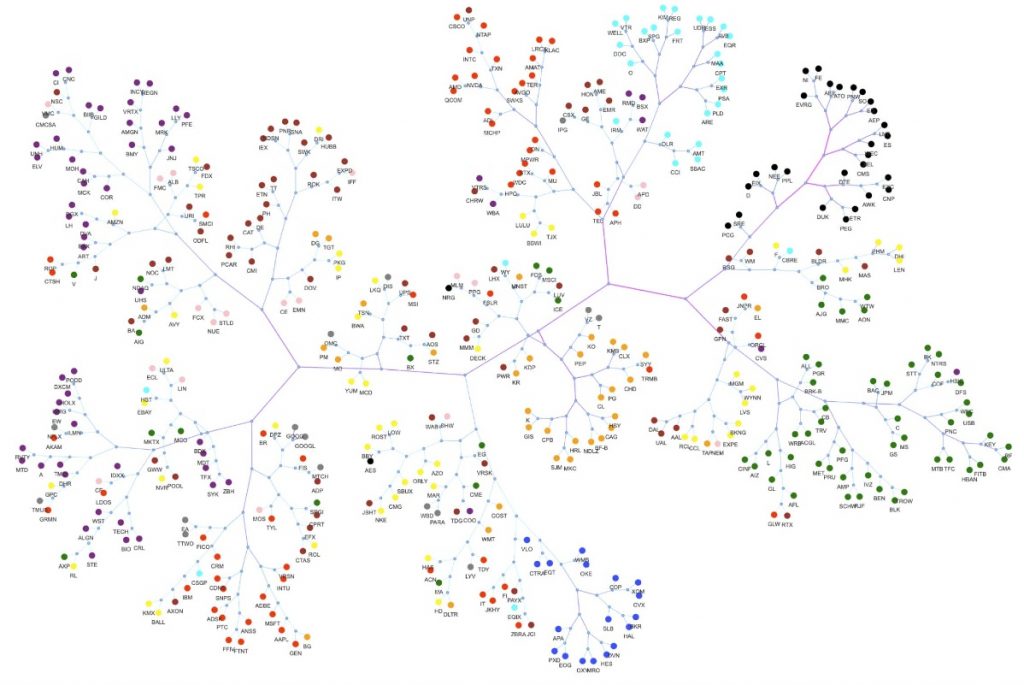
(https://doi.org/10.1088/2632-2153/adc2c7)